How AI is Revolutionizing Digital Twin Creation: From Photos to Perfect 3D Models
- Ritika Chhikara
- Sep 23, 2024
- 3 min read
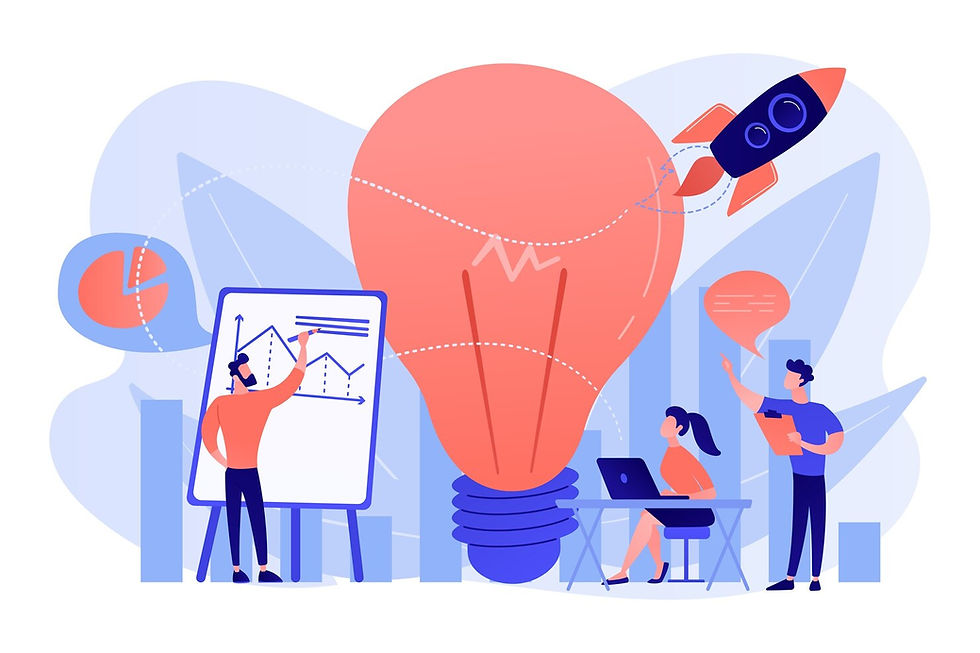
In the rapidly evolving landscape of technology, digital twins have emerged as a game-changer for industries ranging from manufacturing to urban planning. These virtual replicas of physical assets offer a powerful tool for monitoring, analyzing, and optimizing real-world operations. However, the creation of these digital twins has traditionally been a labor-intensive and time-consuming process. Enter artificial intelligence (AI), which is transforming the way digital twins are created, making the process faster, more accurate, and scalable.
The Role of AI in Digital Twin Creation
Digital twins rely on precise 3D models of physical assets, and AI is playing a pivotal role in automating and enhancing the creation of these models. By leveraging AI, industries can now generate detailed 3D models from photographs and other data sources with unprecedented speed and accuracy. This shift is not just about efficiency; it's about enabling new possibilities that were previously unimaginable.
AI-driven technologies are automating key steps in the digital twin creation process, from image alignment to feature detection and texture mapping. This automation reduces human error and significantly cuts down on the time and resources required to generate high-quality 3D models.
AI Photogrammetry: A Game-Changer for 3D Modeling
Photogrammetry, the process of creating 3D models from overlapping photographs, has been revolutionized by AI. Traditional photogrammetry required extensive manual intervention, but AI algorithms now handle many of these tasks automatically.
How AI Enhances Photogrammetry
AI algorithms such as Structure-from-Motion (SfM) and Multi-View Stereo (MVS) are at the forefront of this revolution. These algorithms analyze images to identify key points and generate 3D models. Specifically:
Feature Detection: Algorithms like SIFT (Scale-Invariant Feature Transform) and SURF (Speeded-Up Robust Features) detect key points in images, even in low-texture environments.
Error Reduction: AI minimizes errors caused by lighting variations, occlusions, or poor image quality.
Scalability: AI can process thousands of images simultaneously, making it ideal for large-scale projects like urban planning or infrastructure monitoring.
Automated 3D Reconstruction: The AI Advantage
Traditional 3D reconstruction methods were slow and prone to human error. AI-driven methods, on the other hand, offer significant advantages in terms of speed, accuracy, and scalability.
Traditional vs. AI-Driven 3D Reconstruction
Aspect | Traditional Methods | AI-Driven Methods |
Speed | Slow, manual processes | Fast, automated workflows |
Accuracy | Prone to human error | High precision with AI algorithms |
Scalability | Limited to small-scale projects | Suitable for large-scale applications |
Cost | High labor and equipment costs | Reduced costs with automation |
AI Algorithms in 3D Reconstruction
Deep Learning: Neural networks like Convolutional Neural Networks (CNNs) are used for image segmentation and object recognition.
Generative Adversarial Networks (GANs): These generate high-quality textures and fill in missing data points.
Reinforcement Learning: AI agents optimize drone flight paths for efficient data collection.
Case Studies: AI in Action
Wind Turbine Inspection
AI-powered drones equipped with high-resolution cameras and LiDAR sensors are used to inspect wind turbines. The data is processed using AI algorithms to create digital twins, enabling predictive maintenance and reducing downtime.
Urban Planning
Cities like Rotterdam are using AI-driven photogrammetry to create digital twins of entire urban areas. These models help in simulating traffic patterns, optimizing energy usage, and planning infrastructure projects.
Future Trends in AI-Driven Digital Twin Creation
Generative AI for 3D Models
Generative AI models like World Labs' Large World Models (LWMs) are pushing the boundaries of 3D reconstruction. These models can generate entire virtual environments from minimal input, revolutionizing industries like gaming, robotics, and urban planning.
Integration with IoT and Edge Computing
AI-powered digital twins are increasingly integrated with IoT sensors and edge computing devices, enabling real-time data processing and decision-making. This is particularly useful in industrial settings where latency is critical.
AI-Enhanced Collaboration Tools
Tools like Siemens' Immersive Engineering and NVIDIA Omniverse enable teams to collaborate in real-time within digital twin environments. These platforms use AI to streamline workflows and reduce errors.
Challenges and Opportunities
Challenges
Data Quality: Poor-quality images or scans can lead to inaccurate models.
Computational Costs: High-performance hardware is required for AI-driven 3D reconstruction.
Ethical Concerns: The use of AI in surveillance and data collection raises privacy issues.
Opportunities
Sustainability: AI-driven digital twins can optimize resource usage and reduce environmental impact.
Innovation: New applications in healthcare, entertainment, and education are emerging.
Conclusion
AI is revolutionizing digital twin creation by making the process faster, more accurate, and scalable. From AI photogrammetry to automated 3D reconstruction, these technologies are transforming industries and enabling new possibilities. As AI continues to evolve, the future of digital twins looks brighter than ever, with applications ranging from smart cities to autonomous vehicles.
For industry professionals, staying ahead of these trends is crucial to leveraging the full potential of AI-driven digital twins.
Comments