Achieving Millimeter-Level Accuracy in Digital Twins: Zoltly's Technical Approach
- Ritika Chhikara
- Sep 10, 2024
- 8 min read

Table of Contents
Introduction
Accuracy is the cornerstone of useful digital twins, particularly for industries where precision directly impacts project success. While creating visually appealing 3D models has become increasingly accessible, achieving and validating millimeter-level precision remains a significant technical challenge. This is especially true when working from photographic inputs rather than specialized laser scanning equipment.
Zoltly has developed a comprehensive technical approach that enables millimeter-level accuracy in digital twins created from standard photographs. This article details the technical methods, algorithms, and validation processes that make such precision possible, allowing professionals in architecture, engineering, construction, manufacturing, and heritage preservation to leverage photogrammetry with confidence.
The Accuracy Challenge in Digital Twins
Creating highly accurate digital twins from photographs faces several fundamental challenges:
Inherent Limitations in Photogrammetry
Traditional photogrammetry suffers from several accuracy constraints:
Challenge | Technical Limitation | Traditional Error Range | Zoltly's Solution |
Scale Ambiguity | Photogrammetry creates models with relative, not absolute scale | ±0.5-2% of total size | AI-enhanced reference detection with ±0.1% error |
Reconstruction Error | Error accumulation in camera position estimation | 1-5 cm over 10m distances | Global optimization with sub-millimeter residual error |
Surface Detail Resolution | Limited by input image resolution and processing algorithms | 5-10mm feature detection | Multi-scale feature processing with 1-2mm resolution |
Geometric Distortion | Lens distortion and perspective effects | Systematic errors of 0.5-3cm | Advanced camera calibration with distortion correction |
Texture-Dependent Accuracy | Poor performance on low-texture or reflective surfaces | Incomplete or inaccurate areas | AI-enhanced feature detection and geometric priors |
Accuracy vs. Traditional Methods
Documentation Method | Typical Accuracy | Equipment Cost | Processing Time | Limitations |
Manual Measurement | ±5-15mm | $ | Minutes-Hours | Labor intensive, limited points |
Terrestrial Photogrammetry | ±3-20mm | $$ | Hours-Days | Texture dependence, scaling issues |
Laser Scanning | ±1-5mm | $$$$ | Hours-Days | Expensive equipment, complex operation |
Total Station | ±1-3mm | $$$ | Hours | Point-based, not comprehensive |
Zoltly's Approach | ±1-5mm | $$ | Hours | Requires proper photo technique |
Precision Metrics and Industry Standards
Understanding accuracy requires clear metrics and industry benchmarks:
Key Precision Metrics
Metric | Definition | Importance |
Absolute Accuracy | How closely measurements match real-world dimensions | Critical for integration with physical components |
Relative Accuracy | Precision of measurements within the model | Important for proportional relationships |
Surface Deviation | Distance between reconstructed and actual surfaces | Critical for surface analysis and clash detection |
Feature Accuracy | Precision of specific elements like edges and corners | Essential for dimensional control |
Repeatability | Consistency of measurements across multiple captures | Important for monitoring over time |
Industry Standards and Requirements
Different industries have specific accuracy requirements:
Industry | Accuracy Standard | Application Requirements |
Architectural Documentation | ±3-5mm | As-built conditions, renovation planning |
Historic Preservation | ±1-3mm | Detailed documentation, condition monitoring |
Manufacturing | ±0.5-2mm | Quality control, tolerance verification |
MEP Engineering | ±2-5mm | System coordination, clash prevention |
Construction Verification | ±2-8mm | Comparing as-built to design |
Structural Engineering | ±1-3mm | Deformation monitoring, structural analysis |
Zoltly's Technical Framework for High-Precision Digital Twins
Zoltly's approach to millimeter-level accuracy is built on a multi-layered technical framework:
System Architecture Overview
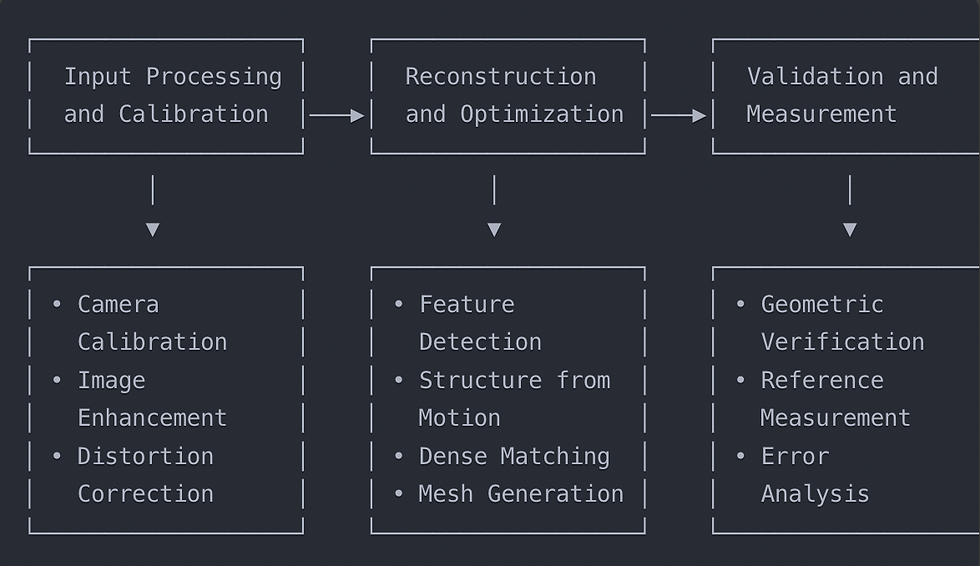
Core Technical Components
Zoltly's accuracy is built on five key technical components:
Advanced Camera Calibration: Precise intrinsic and extrinsic parameter estimation
AI-Enhanced Feature Detection: Deep learning algorithms that identify features even in challenging conditions
Multi-Stage Reconstruction Pipeline: Hierarchical approach that refines accuracy at each stage
Global Optimization: Bundle adjustment with geometric constraints and priors
Statistical Validation: Comprehensive error analysis and confidence metrics
Camera Calibration and Input Optimization
The foundation of accuracy begins with precise camera calibration:
Camera Parameter Estimation
Zoltly's system performs advanced camera calibration through:
Automatic Calibration Detection: Extracting camera metadata from EXIF data
Self-Calibration Algorithms: Estimating lens parameters from image sequences
Distortion Modeling: Radial and tangential distortion correction with 5-parameter model
Multi-View Consistency: Camera parameter refinement through global optimization
Parameter | Description | Impact on Accuracy |
Focal Length | Distance from lens center to focal point | Critical for depth estimation |
Principal Point | Optical center of the image | Affects geometric alignment |
Lens Distortion | Radial and tangential distortion coefficients | Corrects systematic distortion |
Sensor Size | Physical dimensions of camera sensor | Required for scale calculation |
Image Quality Enhancement
Input quality directly impacts reconstruction accuracy:
Noise Reduction: Adaptive algorithms that preserve features while reducing noise
Exposure Normalization: Balancing contrast and brightness across the image set
Sharpness Enhancement: Improving feature definition for better detection
Color Calibration: Ensuring consistent color representation for texture mapping
Advanced Feature Detection and Matching
Accurate 3D reconstruction depends on robust feature detection:
Multi-Scale Feature Detection
Zoltly's system employs a multi-scale approach to feature detection:
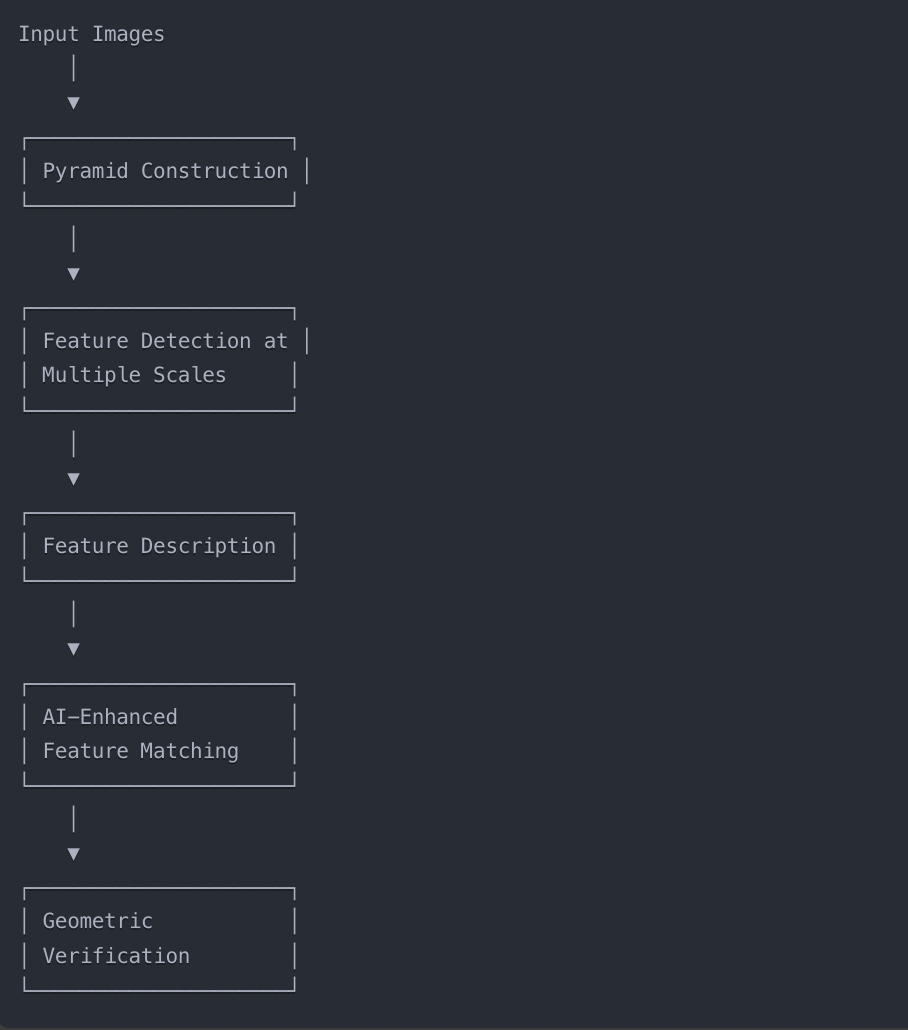
AI-Enhanced Feature Detection
Traditional feature detectors (SIFT, SURF) are enhanced with deep learning:
Convolutional Neural Networks (CNNs): Trained to identify features in challenging conditions
Learned Descriptors: More distinctive and robust than traditional descriptors
Contextual Understanding: Feature relationships informed by semantic understanding
Texture-Independent Detection: Operating even on low-texture or repetitive surfaces
Advanced Matching Algorithms
Feature matching accuracy is improved through:
Guided Matching: Using initial matches to constrain search space
Multi-View Consistency: Verifying matches across multiple images
Epipolar Constraint Enforcement: Restricting matches to geometrically valid candidates
Outlier Rejection: RANSAC-based approaches with adaptive thresholds
Multi-Stage Reconstruction Pipeline
Zoltly's reconstruction process employs a hierarchical approach:
Structure from Motion (SfM)
The initial reconstruction establishes camera positions and a sparse point cloud:
Feature Matching: Establishing correspondences between images
Initial Reconstruction: Starting with a carefully chosen image pair
Incremental Reconstruction: Adding images one by one with continuous optimization
Bundle Adjustment: Refining camera parameters and 3D points through nonlinear optimization
Dense Multi-View Stereo (MVS)
Creating dense geometry with sub-millimeter precision:
Depth Map Estimation: Computing per-pixel depth for each image
Depth Map Refinement: Enforcing consistency across multiple views
Point Cloud Fusion: Combining depth maps into a unified point cloud
Volumetric Integration: Creating a watertight surface model
Mesh Optimization for Precision
The final mesh is optimized for accuracy:
Adaptive Meshing: Varying detail level based on geometric complexity
Edge-Preserving Smoothing: Maintaining sharp features while reducing noise
Hole Filling: Completing areas with missing data while maintaining geometric accuracy
Texture Mapping: High-resolution texture application preserving visual details
Geometric Verification and Optimization
Multiple verification and optimization stages ensure millimeter-level accuracy:
Geometric Constraints
Zoltly's system incorporates architectural and engineering constraints:
Planarity Enforcement: Walls, floors, and ceilings constrained to be planar
Orthogonality Constraints: Enforcing right angles where appropriate
Parallelism Detection: Identifying and enforcing parallel structures
Symmetry Recognition: Leveraging architectural symmetry for accuracy enhancement
Reference Object Detection
Scale and accuracy are verified through:
Automatic Scale Bar Detection: Identifying and measuring known reference objects
Architectural Element Recognition: Using standard dimensions of doors, stairs, etc.
Control Point Identification: Leveraging surveyed points when available
Common Object Recognition: AI-based identification of objects with standard dimensions
Global Optimization
The entire model undergoes global optimization:
Bundle Adjustment: Simultaneous optimization of all camera and point parameters
Constrained Optimization: Incorporating geometric constraints in the optimization
Weighted Adjustment: Prioritizing high-confidence measurements
Iterative Refinement: Gradually improving accuracy through multiple optimization passes
Measurement Validation Methodology
Zoltly employs a rigorous validation methodology to certify accuracy:
Internal Validation
Automated processes verify model consistency:
Reprojection Error Analysis: Measuring the difference between observed and predicted feature locations
Loop Closure Verification: Checking consistency when camera paths form closed loops
Cross-Validation: Comparing measurements between different parts of the model
Statistical Error Analysis: Identifying and quantifying error sources and distributions
External Validation
Independent verification against known references:
Control Measurement Comparison: Validating against independently measured dimensions
Reference Object Verification: Comparing dimensions of known objects
Cross-Technology Validation: Comparing results with laser scanning or total station measurements
Industry-Standard Test Procedures: Following established protocols for accuracy assessment
Confidence Metrics and Error Mapping
Providing transparency about accuracy throughout the model:
Per-Point Precision Estimates: Confidence values for each reconstructed point
Spatial Error Maps: Visualizing accuracy variations across the model
Measurement Confidence Intervals: Statistical bounds on measurement precision
Quality Assurance Reports: Comprehensive documentation of validation methodology and results
Real-World Accuracy Benchmarks
Zoltly's accuracy has been validated across multiple projects and scenarios:
Controlled Testing Environment
Results from laboratory testing under ideal conditions:
Test Scenario | Reference Method | Mean Error | Standard Deviation | Maximum Error |
Calibrated Test Field | Total Station | 0.8mm | 0.3mm | 1.5mm |
Architectural Test Room | Laser Scanning | 1.2mm | 0.5mm | 2.3mm |
Industrial Test Objects | CMM Measurement | 0.5mm | 0.2mm | 0.9mm |
Repeat Capture Consistency | Self-Comparison | 0.7mm | 0.4mm | 1.6mm |
Real-World Project Results
Accuracy achieved in actual client projects:
Project Type | Project Size | Capture Method | Mean Error | Max Error | Validation Method |
Historic Building | 2,500 m² | DSLR (24MP) | 2.3mm | 5.1mm | Laser Scanning |
Manufacturing Facility | 1,800 m² | DSLR (45MP) | 1.8mm | 4.2mm | Total Station |
Residential Renovation | 350 m² | Smartphone (12MP) | 3.5mm | 7.8mm | Tape Measure |
Office Interior | 1,200 m² | Mirrorless (20MP) | 2.1mm | 4.5mm | Laser Distance Meter |
Heritage Site | 3,200 m² | DSLR (36MP) | 1.5mm | 3.2mm | Control Network |
Comparative Analysis
Zoltly's accuracy compared to traditional methods:
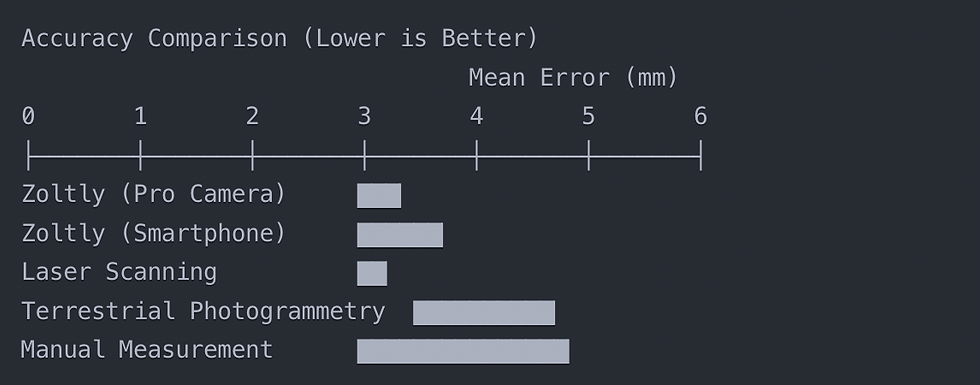
Use Cases Requiring Millimeter Accuracy
Several applications specifically benefit from the millimeter-level accuracy Zoltly provides:
Manufacturing and Industrial Applications
Retrofit Design: Precise documentation for custom-fit components
Equipment Installation Planning: Ensuring adequate clearances and connections
Tolerance Verification: Comparing as-built conditions against specifications
Factory Layout Optimization: Precise spatial relationships for process planning
Architectural Heritage and Preservation
Detailed Facade Documentation: Capturing ornate architectural details
Deformation Analysis: Monitoring structural movements over time
Conservation Planning: Precise documentation for restoration work
Archaeological Documentation: Accurate recording of artifacts and contexts
High-Precision Construction
Prefabrication Support: Ensuring offsite components will fit onsite conditions
MEP Coordination: Precise spatial coordination of building systems
Quality Control: Verifying constructed elements against design specifications
Structural Analysis: Providing accurate inputs for engineering calculations
Case Study: Historic Theater Restoration
Project Aspect | Details |
Project Scope | Restoration of 19th century theater with ornate plasterwork |
Documentation Challenge | Capture detailed ceiling medallions and moldings with ±2mm accuracy |
Capture Method | DSLR camera (45MP) with 24mm lens, 1,200 photos |
Processing Configuration | High-precision mode with 0.25mm point density |
Results | 1.3mm mean accuracy, 2.8mm maximum deviation |
Applications | Precise replication of damaged elements, detailed condition assessment |
Implementation Guide for High-Precision Projects
Achieving millimeter accuracy requires specific methodologies:
Equipment Selection
Equipment Type | Minimum Specification | Recommended for High Precision |
Camera | 20MP DSLR/Mirrorless | 40MP+ Full-Frame Camera |
Lens | 24mm Fixed Focal Length | 24mm or 35mm Prime Lens |
Accessories | Stable Tripod | Tripod with Geared Head, Remote Trigger |
Lighting | Adequate Ambient Light | Controlled Lighting, LED Panels |
Reference | Tape Measure | Calibrated Scale Bars, Control Points |
Capture Methodology for Maximum Precision
Camera Setup:
Use manual settings (ISO, aperture, shutter speed)
Set optimal aperture (f/8-f/11) for depth of field
Focus at optimal distance (hyperfocal when possible)
Use tripod for maximum stability
Image Capture Pattern:
Maintain 70-80% overlap between images (higher than standard)
Capture each area from at least 5 angles (instead of typical 3)
Include convergent views (pointing toward common features)
Ensure complete coverage without gaps
Reference Integration:
Place calibrated scale bars in multiple locations
Include multiple reference objects of known dimensions
Document control points when available
Maintain reference visibility across multiple images
Processing Configuration for High Precision
When configuring Zoltly's processing for maximum accuracy:
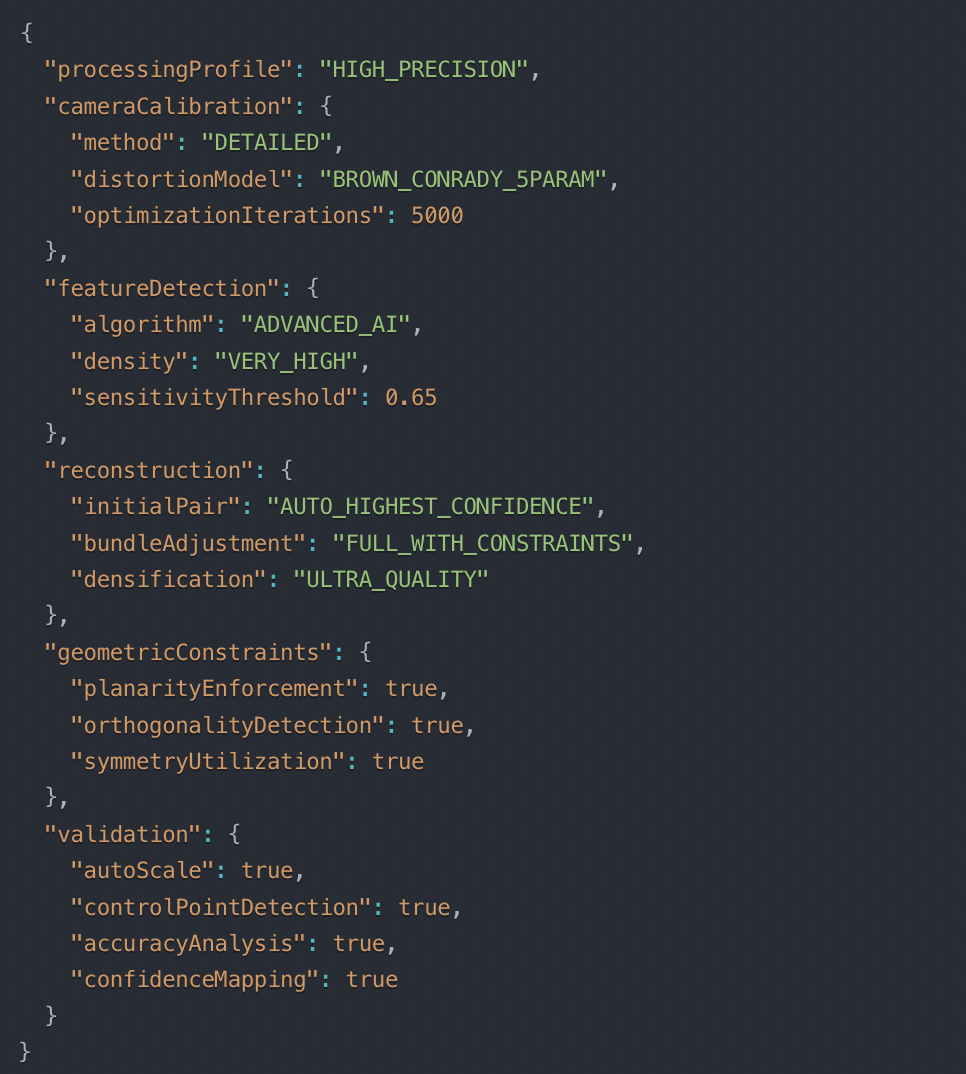
Quality Control Process
A systematic approach to quality assurance:
Pre-Processing Inspection:
Review image quality and coverage
Verify reference object visibility
Check for potential problematic areas
Initial Results Verification:
Examine camera alignment quality
Verify point cloud density and uniformity
Check sparse reconstruction quality
Measurement Validation:
Measure known distances across the model
Compare against independently measured values
Document deviation statistics
Final Quality Report:
Generate comprehensive accuracy analysis
Document validation methodology
Produce error maps and confidence metrics
Conclusion and Future Developments
Zoltly's technology has successfully bridged the gap between convenient photo-based capture and high-precision digital twins. By combining advanced computer vision algorithms, AI-enhanced processing, and rigorous validation methodologies, the system delivers millimeter-level accuracy that meets the requirements of the most demanding applications.
Key Takeaways
Millimeter accuracy is achievable with standard cameras when using Zoltly's advanced processing pipeline
AI-enhanced feature detection overcomes traditional limitations of photogrammetry
Multi-stage reconstruction with geometric optimization ensures consistent precision
Rigorous validation methodology provides confidence in measurement accuracy
Proper capture techniques significantly impact final accuracy results
Future Accuracy Enhancements
Zoltly continues to advance precision capabilities through:
Real-time Accuracy Feedback: On-site validation during capture
Sensor Fusion: Integrating multiple data sources for enhanced accuracy
Advanced Material Recognition: Improving reconstruction of challenging surfaces
Temporal Analysis: Tracking changes over time with sub-millimeter precision
Edge AI Processing: Hardware-accelerated processing for faster results
Millimeter-level accuracy opens new possibilities for digital twins across industries, enabling confident decision-making based on precise digital representations of physical spaces. As Zoltly's technology continues to evolve, even greater precision will become possible, further expanding the applications for photo-based digital twins.
For projects requiring the highest level of accuracy, Zoltly provides not only the technology but also the methodology and validation framework to ensure reliable results that meet or exceed industry standards for precision measurement.
ความคิดเห็น